Data quality dimensions – from Accuracy to Uniqueness
Gathering data for KPI results is one of the most common challenges that professionals face when measuring performance. An effective data gathering process should not only provide timely performance data, but also highly qualitative data. Designing such a process is indeed a challenge in itself, and establishing what quality data means is also a demanding task.
Data quality dimensions can differ from one company to another, as they indicate what characteristics are important in order to evaluate a set of data as being at the desired standards. There are a variety of features that can be explored, such as:
- Accuracy;
- Auditability;
- Completeness;
- Conformity;
- Consistency;
- Coverage;
- Duplication;
- Integrity;
- Security;
- Specifications;
- Timeliness;
In practice, when collecting data for KPIs, only 3 to 6 characteristics are selected as criteria for evaluating data quality. In this context, I will present more details for some of the most popular data quality dimensions.
1. Accuracy – it indicates the extent to which data reflects the real world object or an event.Examples:
- The temperature shown by the thermometer is accurate if it is the same with the real temperature;
- The addresses from the client datadase are accurate when they indicate the real location of customers;
Inaccuracy can be reflected by incorrect values, whether numbers or descriptive data (gender, location, preferences etc.) or other information that is not updated.
2. Completenesss – it refers to whether all available data is present. When data is due to unavailablity, this does not represent a lack of completeness.Examples:
- When performance data for $ Sales is required for the last six month, but results are submitted for the last five months only;
- Customer details repository consists in name, surname, address and email. However, data for surname is missing in more than one client, even if this infomation should be available.
Examples:
- An employee status is terminated but his pay status is still active;
- There are sales registered in January, but no orders registered in that month.
Examples:
- Possible values for % Transaction processed are from +0% to +100%, the data for this KPI cannot be an absolute or negative value;
- For customers gender there are only two possible values: Feminin and Masculin
6. Uniqueness – points out that there should be no data duplicates reported. Each data record should be unique, otherwise the risk of accessing outdated information increases. For example, we may have in our database two customers that were registered as Tom Adams and Thomas Adams, which in fact are the same person, but the latter has the latest details. Now this situation poses the risk that a Customer Service representative may access outdated information under Tom Adams and will not be able to contact the client.
We have to keep in mind that these dimensions are not always 100% met, meaning that data can be accurate but incomplete, or it can meet all 5 criterias except for timeliness. As managers have to make decisions based on data, it is very important to perform a short audit of data before compiling KPI results in a performance report, based on the quality dimensions presented above. Therefore, if data is not complete or there is an uniqueness issue, data users must be informed in order to keep this in mind when deciding.
References:
- Execution-MiH (n.d.), Data Quality Definition- What is Data Quality?
- Melissa Data (n.d.), 6 Key Data Quality Dimensions
Image source:
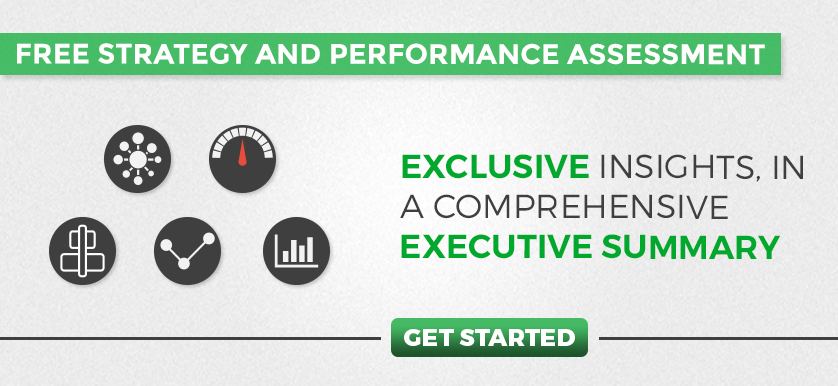
Tags: Data analysis, operational performance