7 Key Steps To Build a Data Team From Scratch
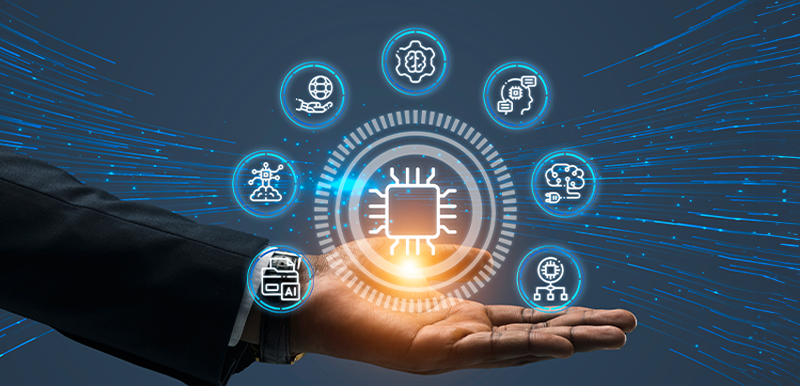
Despite the continuous hype around data analytics and the rapid acceleration of data technologies such as machine learning (ML) and artificial intelligence (AI), most companies are lagging behind with low data capabilities and no in-house data team in place. These companies have their data either fully unleveraged or marginally analyzed by executives on the side of their jobs to produce limited reports.
In such a situation, pushing the organization up the hill of data maturity would require building a team of data-specialized personnel. Building such a team can be daunting, as every company would have different conditions and no one way can fit all cases. However, covering the following main grounds can help cut miles on the road to building a data team from the ground up.
First, nurture the environment and plant the seeds. Data teams cannot grow in a vacuum. To prepare the organization to become data-driven with a data team, enhancing the organizational data culture is a good starting point. Having employees at all levels with a data-driven mentality and an understanding of the role of data analytics can significantly prepare the room for the planned team.
Second, connect with stakeholders and recognize priority needs. Carrying out data culture programs inside the organization can open up opportunities to have meaningful discussions with stakeholders on different levels about their data needs, what they already do with data, and what they want to achieve, in addition to having better insights into the pre-existing data assets. This is a good stage to recognize the organization’s data pain points, which would then be the immediate and strategic objectives of the future data team.
Third, define the initial structure of the team. According to the scale of the organization and the identified needs, data teams can have one of three main structures:
- Centralized: This involves having all data roles within one team reporting to one head, chief data officer (CDO), or a similar role. All departments in the organization would request their needs from the team. This is a straightforward approach, especially for small-size companies, but can end up in a bottleneck if not scaled up continuously to meet the organization’s growing needs.
- Decentralized: This requires disseminating all data roles and infusing them into departmental teams. This mainly aims to close the gap between technical analysis and business benefits as analysts in every team would be experts in their functional areas. However, the approach may lead to inconsistencies in data management and fragile data governance.
- Hybrid: This consists of having governance, infrastructure, and data engineering roles within a core team, along with embedding data analysts, business analysts, and data scientists in departmental teams. The allocated personnel would report to the respective department head as well as the data team head. This approach combines the benefits of both centralized and decentralized structures and is usually applicable in large organizations as they require more headcount in their data teams.
Read More: How Data Analytics Can Improve Company Performance
Fourth, map the necessary tech stack and data roles. As the previous stages have uncovered the current uses and needs of data in an organization, it should be easier to start figuring out the tech tools that the team would be initially working with. Mapping the needed tech stack would be the first pillar before moving on to the hiring process. The second pillar would involve defining the roles that the team would need in its nascent stage to meet the prioritized objectives.
Several data job titles can be combined in a data team, with many of them having specializations that intersect with or bisect each other. However, there are three main role areas that should be considered for starting data teams:
- Data engineering: implementing and managing data storage systems, integrating scattered datasets, and building pipelines to prepare data for analysis and reporting
- Data analysis: performing final data preparation and extracting main insights to inform decision-making
- Data science: building automated analysis and reporting systems, usually concerned with predictive and prescriptive machine learning models
Fifth, follow step-by-step team recruitment. Hiring new employees for the data team is one option. The other option can be upskilling existing employees with an interest in a data career and with minimum required skills. Even employees with just interest and no minimum required skills can be reskilled to fill some roles, especially within an initial data team.
The team does not need to take off with full wings. It can start small and gradually grow. Typically, data teams would start with data analysts who have extra skills in data engineering, data engineers who have experience with ad-hoc analyses and reporting, or a limited combination of both. In later stages, other titles can join onboard.
The baby-step-building approach is more convincing for stakeholders as it can be more efficient from a return-on-investment (ROI) perspective. Starting with a full-capacity team may end up being too costly for the organization, which could lead to the budding project being cut off in its prime.
Read More: Why is Data Integration Important and How Can We Achieve It?
Sixth, deliver ad-hoc analyses, heading towards long-term projects. In the beginning, data analytics experts at the organization would be expected to answer random requests and solve urgent data-related problems, like developing quick reports and reporting on-spot metrics. This is a good point to prove how data personnel can be of direct benefit to the organization.
However, along with delivering said ad-hoc requests, the data team should have strategic goals to enhance and develop the overall data maturity of the organization, like organizing, integrating, and automating the analytics processes and installing advanced predictive models. These long-term projects should foster the organization’s data maturity, which should result in ad-hoc requests being less frequent as all executives should be self-sufficient in using the installed automated reports and systems. In such a data-mature environment, the team would have time to advance their data products continuously, opening up new benefit opportunities.
Seventh, fortify the team’s presence. Strategic projects with shorter implementation periods and more immediate impact should be prioritized over longer ones, especially in the beginning. That would help continuously prove the benefits of the data team and the point of its foundation. Owning the products of the data team by having its name on it can help remind decision-makers of the team’s benefit. In addition, it is highly useful for the data team’s head to have access to top managerial levels to keep promoting the team’s presence and expansion.
Building a data team from scratch requires careful planning, investment, and commitment from organizational leadership. By following these guidelines and adapting them to their specific needs, organizations without prior data capabilities can establish a robust data team capable of driving innovation and offering a competitive advantage through data-driven insights.
Learn more about data management by exploring our articles on data analytics.
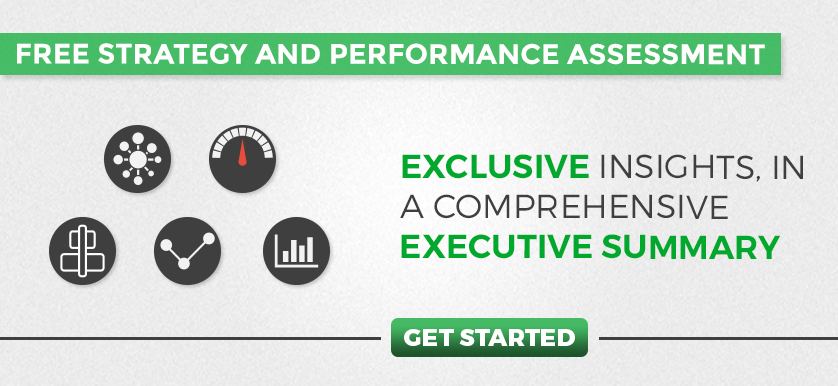
Tags: analytics team, data, data analytics, data team